AI-Powered Thermal Control of EV Batteries
Electric vehicle battery packs operate under dynamic thermal conditions, with cell temperatures fluctuating between -20°C and 45°C during normal operation. These thermal variations, combined with aging effects and charging cycles, create complex patterns that influence both immediate performance and long-term degradation. Traditional fixed-threshold monitoring systems often fail to capture the nuanced relationships between operational parameters and thermal behavior.
The fundamental challenge lies in developing predictive thermal management systems that can anticipate temperature changes across multiple time scales while accounting for cell-to-cell variations and degradation states.
This page brings together solutions from recent research—including time-varying twin converter models for thermal failure prediction, hybrid thermal-neural network approaches for temperature estimation, spatio-temporal learning frameworks for real-time monitoring, and aging-aware temperature forecasting systems. These and other approaches focus on implementing robust thermal management strategies that can be deployed in production vehicles while maintaining computational efficiency.
TABLE OF CONTENTS
1. Hybrid Physical-Data Models for Temperature Prediction
Predicting temperature behavior in electric vehicle (EV) batteries presents unique challenges due to the complex, nonlinear thermal dynamics of lithium-ion cells. As battery technology evolves, researchers have increasingly turned to hybrid approaches that combine physical models with data-driven techniques to achieve greater accuracy and reliability.
One innovative solution developed by researchers combines a physical battery model with a Long Short-Term Memory (LSTM) neural network to predict potential thermal runaway events. This hybrid system integrates an electrothermal coupling model that accounts for internal temperature, state of charge (SOC), and equivalent circuit parameters. The LSTM network, trained on real-time voltage, current, and surface temperature data, forecasts temperature trends while a diagnostic module analyzes these predictions to issue early warnings. What sets this hybrid prediction model apart is its ability to provide improved accuracy and early anomaly detection using only readily available sensor data, making it particularly suitable for real-time applications in commercial EV battery management systems.
For large-format laminated batteries, which are increasingly popular in EVs due to their high energy density and compact packaging, thermal management presents additional challenges. These batteries frequently develop localized hot spots and temperature non-uniformity resulting from structural and manufacturing inconsistencies. To address these issues, researchers have developed a thermal-neural network coupling model that combines physics-based thermal modeling with neural network capabilities. This approach captures complex thermal dynamics across wide temperature ranges while reducing dependence on extensive sensor networks. The integration of interpretable thermal models with the adaptive capabilities of neural networks enables high estimation accuracy under real-world driving conditions, making it well-suited for onboard implementation.
The interdependence between state of charge and temperature becomes particularly critical under high-current operations and extreme temperature scenarios. Recognizing this relationship, a third approach introduces an electric-thermal-neural network (ETNN) coupling model that integrates electrical and thermal models with neural networks. This synergistic approach enhances estimation accuracy across broad operating ranges while eliminating the need for hardware-intensive solutions like electrochemical impedance spectroscopy. The ETNN framework supports robust, real-time estimation that enables safer and more efficient battery operation under dynamic conditions.
Recent advances in deep learning architectures have led to the development of a Transformer-based model for real-time prediction of thermal failures in EV batteries. This system converts multivariate signals (temperature, voltage, current) into time-series features and processes them through a temporal attention mechanism. The time-varying twin converter model excels at capturing both global patterns and localized signal variations, enabling earlier and more accurate prediction of thermal failures. Its compatibility with standard EV hardware platforms and ability to provide online monitoring make it a practical solution for next-generation battery thermal management systems.
2. Spatiotemporal Learning and Temperature Field Reconstruction
A fundamental limitation in EV battery thermal management is the inability to directly monitor internal temperatures with sufficient spatial resolution. Physical constraints and cost considerations typically restrict manufacturers to using only a handful of sensors, creating significant blind spots in thermal monitoring. Traditional computational fluid dynamics (CFD) approaches attempt to fill these gaps but are often too computationally intensive for real-time applications and may lack accuracy due to simplified thermodynamic models.
Researchers at several institutions have developed novel approaches to overcome these limitations. One breakthrough method combines compressed sensing and LSTM neural networks to reconstruct complete internal temperature fields using minimal sensor inputs. This technique learns the complex relationship between sparse sensor data and actual temperature distribution, allowing it to estimate temperatures at unsensed locations with remarkable accuracy. The temporal capabilities of LSTM networks enable the system to track dynamic temperature changes during variable load conditions, providing continuous thermal monitoring with significantly reduced hardware complexity.
Building on these advances, another team has developed a 3D-CNN-based spatiotemporal learning framework that reconstructs corrected three-dimensional temperature fields from limited sensor readings. This approach integrates not only temperature, voltage, and current data but also incorporates physical parameters such as internal resistance and heat generation mechanisms. Drawing inspiration from generative modeling techniques, the system uses measured data as constraints to produce physically consistent thermal maps. A key innovation in this framework is the integration of Kalman filtering within the training loop, which substantially improves temporal prediction by accounting for system noise and dynamic changes.
What distinguishes this 3D-CNN approach from previous methods is its ability to preserve spatial correlations across the entire battery pack. This capability is particularly valuable in complex battery geometries where thermal gradients can vary significantly between cells. By embedding physical understanding into deep learning architectures, both approaches effectively bridge the gap between sensor limitations and the need for comprehensive thermal monitoring.
These AI-driven spatiotemporal learning techniques represent a paradigm shift in thermal management, transforming sparse sensor data into rich, actionable information. The resulting high-resolution thermal field reconstructions enable more precise control strategies, enhance safety through early detection of thermal anomalies, and open new possibilities for predictive diagnostics in next-generation EV battery systems.
3. Reinforcement Learning for Thermal Management Control
Traditional thermal management systems (TMS) in electric vehicles operate on fixed logic rules and pre-defined control parameters, creating inherent limitations in their ability to adapt to changing conditions. These conventional systems struggle to balance battery safety with energy efficiency, particularly during dynamic driving scenarios where thermal loads can change rapidly and unpredictably.
To address these limitations, researchers have turned to reinforcement learning (RL), a branch of machine learning particularly well-suited for sequential decision-making problems. One innovative approach employs offline reinforcement learning with a Decision Transformer architecture to manage battery pack temperatures adaptively. This system learns optimal cooling and heating actions from large-scale historical driving data, enabling it to predict appropriate thermal responses based on real-time battery states. What makes this approach particularly effective is its sophisticated reward function design, which balances temperature deviation penalties against energy usage from water pumps and coolant adjustments. By incorporating stratified sampling techniques and training on real-vehicle test data, the system achieves remarkable adaptability across diverse operating conditions.
Another significant advancement combines predictive and reactive strategies through the integration of supervised learning with reinforcement learning. This hybrid approach first forecasts future battery temperatures using historical and current data, then applies a reinforcement learning model to select optimal cooling actions based on both predicted and real-time thermal states. The system executes these actions through a fuzzy control algorithm, allowing for fine-grained thermal adjustments in immersed liquid-cooled battery systems. The real-time predictive control capabilities enable proactive interventions before critical temperature thresholds are reached, significantly enhancing battery safety and longevity.
For more complex vehicle systems, researchers have developed Q-learning approaches that optimize thermal management holistically across the battery, motor, and passenger cabin. Unlike isolated control systems, this integrated approach continuously learns from current vehicle status and power demands to compute optimal heating allocations across all subsystems. The real-time adaptability allows the system to respond effectively to unknown or changing operating conditions, maintaining thermal equilibrium across multiple vehicle components simultaneously. This unified control strategy represents a significant step forward in achieving system-wide thermal optimization that enhances both energy efficiency and passenger comfort.
These reinforcement learning approaches share a common advantage: they can discover control strategies that might not be obvious to human engineers, particularly for complex, multi-objective optimization problems where traditional rule-based systems fall short. As EV manufacturers continue to push the boundaries of battery performance and fast-charging capabilities, these adaptive, learning-based thermal management systems will likely become increasingly essential for maintaining battery health and safety.
4. Predictive and Model Predictive Control (MPC) for Thermal Regulation
The dynamic nature of EV operation presents significant challenges for thermal management systems, which must balance battery health, energy efficiency, and performance across widely varying conditions. Model Predictive Control (MPC) has emerged as a powerful framework for addressing these challenges, offering advantages over traditional control methods through its ability to anticipate future states and optimize control actions accordingly.
One innovative MPC approach integrates vehicle speed prediction within the control framework to dynamically adjust cooling temperature setpoints. This model predictive control (MPC) system responds to real-time weather conditions and predicted vehicle speed, enabling robust temperature regulation even during transitional seasons when cabin HVAC systems might be inactive. The multi-objective optimization strategy simultaneously minimizes cooling energy consumption while mitigating battery thermal aging—a critical factor in long-term battery health. By accounting for cumulative thermal stress rather than just instantaneous temperature, this system significantly enhances battery longevity compared to conventional on/off control strategies.
Computational intensity has traditionally limited the real-time application of MPC in vehicle systems. To overcome this barrier, researchers have developed a two-stage planning algorithm with linearized system modeling. This thermal management control system incorporates forecasted driving conditions, energy efficiency ratios, and state estimations of both the battery and passenger compartment to determine optimal power output sequences and coolant flow rates. The system translates optimization results into operational commands through a PID controller that manages components such as compressors and flow valves. A particularly innovative aspect is the use of particle swarm optimization (PSO) to fine-tune thermal parameters, effectively balancing temperature uniformity with energy consumption.
Cold climate operation presents unique thermal challenges due to the absence of waste heat from internal combustion engines. A distributed MPC architecture addresses this through cooperative heating control between the passenger compartment and battery. By decomposing the thermal system into two subsystems—each managed by a dedicated MPC controller—the method enables real-time, multi-input, multi-output optimization. The cabin and battery subsystems operate using a heat pump system and PTC heaters, with state-space models driving a rolling horizon optimization process. Environmental sensors provide continuous feedback, ensuring adaptive control across changing conditions.
Driver behavior also significantly impacts thermal management requirements. Recognizing this, researchers have developed systems that utilize driver intention recognition to proactively manage battery temperature. This approach uses fuzzy logic to infer acceleration and braking intent from pedal behavior, vehicle speed, and ambient conditions. By predicting future power demand and associated battery heating, the system makes anticipatory adjustments to cooling parameters such as electronic water pump flow rates. This proactive strategy reduces thermal lag, prevents temperature overshoots, and enhances both safety and battery lifespan.
These MPC approaches represent a significant evolution beyond reactive thermal management, enabling systems that can anticipate needs, optimize across multiple objectives, and adapt to both environmental conditions and driver behavior. As computational capabilities in vehicles continue to advance, these predictive control strategies will likely become standard features in EV thermal management systems.
5. Aging-Aware and Degradation-Informed Temperature Forecasting
Battery aging significantly alters thermal behavior in lithium-ion cells, rendering conventional temperature prediction models increasingly inaccurate as batteries degrade. This relationship between aging and thermal response creates a critical challenge for long-term battery management in electric vehicles.
Traditional thermal models typically assume consistent battery properties throughout the battery's lifespan—an assumption that fails to account for the progressive changes in internal resistance, capacity, and thermal conductivity that occur with cycling and calendar aging. To address this limitation, researchers have developed a clustering-based approach that segments battery data according to aging levels. This method applies a Temporal Convolutional Network (TCN) to each cluster, capturing aging-specific thermal dynamics with greater precision. The outputs feed into an Online Sequential Extreme Learning Machine (OSELM) for real-time forecasting, with performance enhanced through an Enhanced Honey Badger Algorithm (XHBA). The algorithm employs Chebyshev chaotic mapping and Levy flight strategies to optimize the model parameters, significantly improving prediction accuracy for batteries at different stages of degradation.
Another challenge in aging-aware thermal management is the difficulty of obtaining accurate physical parameters as batteries degrade. A data-driven temperature forecasting method addresses this by operating without requiring exhaustive physical characterization. The approach uses historical data from multiple battery cells to train two neural networks: one for predicting future current profiles and another for forecasting temperature based on both historical and predicted parameters. The cross-cell data utilization framework leverages information from different cells to enhance prediction accuracy, while a statistical correction mechanism improves reliability under complex operating conditions. By eliminating the need for hard-to-measure inputs like time-varying internal resistance, this solution offers a practical alternative to traditional thermal modeling for aging batteries.
The relationship between aging and thermal behavior extends to state-of-charge (SoC) estimation, where degradation introduces inconsistencies in voltage and current profiles. Researchers have developed a system that integrates K-Nearest Neighbors (KNN) learning with a neural network circuit to monitor and predict cell aging in real time. The temperature-aware SoC estimation mechanism accounts for temperature-dependent degradation patterns, enabling early identification of aging-induced anomalies and supporting safer thermal operation throughout the battery's service life.
These aging-aware approaches represent a crucial advancement in thermal management for EV batteries, acknowledging that thermal behavior evolves throughout a battery's lifecycle. By incorporating degradation effects into prediction models, these systems maintain accuracy and reliability even as batteries age, ultimately extending useful life and enhancing safety for electric vehicles in long-term operation.
6. Virtual Sensors and Indirect Temperature Estimation
Direct temperature measurement within EV battery packs faces significant practical limitations. Physical sensors can only be placed in accessible locations, typically on cell surfaces or between cells, leaving internal temperatures—often the most critical for safety and performance—unmeasured. Additionally, extensive sensor networks increase system cost, complexity, and potential points of failure.
To overcome these constraints, researchers have developed virtual sensing techniques that estimate temperatures at inaccessible locations using models and limited physical measurements. One approach constructs a virtual sensor-based temperature prediction system that simulates battery thermal behavior during charge-discharge cycles. By fusing real-time sensor data with simulation outputs and applying dimensionality reduction techniques like Principal Component Analysis (PCA) alongside cyclic neural networks, this method enables dynamic temperature forecasting with minimal hardware. The system continuously adapts to changing battery conditions, maintaining accuracy while significantly reducing sensor count and associated costs.
Thermal imbalance presents another challenge, as uneven heat generation creates significant temperature gradients between battery cores and surfaces. A model-based estimation framework addresses this by constructing an equivalent thermal network of the battery system. The approach uses offline testing data to calibrate the network parameters and integrates real-time operational data to estimate internal temperatures that cannot be directly measured. A Luenberger observer provides feedback correction, continuously refining the internal state vector to enhance estimation accuracy over time. This model effectively captures spatial heterogeneity in temperature distribution and can compensate for sensor failures, making it particularly valuable for integration into production vehicle Battery Management Systems (BMS).
For multi-cell battery packs, where thermal behavior may vary significantly between cells, researchers have developed a deep learning-based monitoring method that improves anomaly detection granularity. This system addresses the complex thermal dynamics of hard-packed lithium batteries through sophisticated feature engineering. It employs kernel PCA for dimensionality reduction and homologous dimension expansion to enhance feature abstraction, with dynamic weighting and fusion to adapt to operational changes. The processed data vectors undergo analysis by a pre-trained model that detects anomalies and generates cell-specific handling strategies.
A particularly promising approach combines machine learning with physical modeling in a hybrid thermal diagnosis framework. This hybrid prediction framework integrates a gated recurrent unit (GRU)-based neural network with a state-space model that estimates internal battery parameters. The GRU forecasts serve as observations for an Adaptive Unscented Kalman Filter (AUKF), which fuses them with the physical model to generate next-step predictions. This dual approach enhances robustness against noise and model uncertainties, enabling reliable early detection of thermal risks.
These virtual sensing techniques represent a significant advancement in battery thermal management, transforming limited direct measurements into comprehensive thermal awareness. By reducing hardware requirements while maintaining or even improving monitoring capabilities, these approaches enable more cost-effective, reliable, and sophisticated thermal management in production electric vehicles.
7. Route-, Navigation-, and Driving Behavior-Based Thermal Management
Traditional battery thermal management systems operate reactively, activating heating or cooling only after battery temperatures cross predefined thresholds. This approach fails to leverage valuable contextual information about upcoming driving conditions, resulting in inefficient energy usage and potentially compromised battery longevity.
Vehicle navigation systems contain rich predictive information about route topology, traffic conditions, and environmental factors that directly influence battery thermal loads. Researchers have developed an AI-driven thermal management system that harnesses this data to proactively regulate battery temperature. The system forecasts thermal states based on route characteristics, navigation data, and driver-specific behavior patterns. This predictive thermal management system integrates real-time GPS data, route information, weather conditions, and driver profiles to make informed decisions about thermal control activation. If the predicted battery temperature remains within safe thresholds throughout the planned journey, the system conserves energy by minimizing thermal control operations. Conversely, when extended exposure to critical temperature ranges is anticipated, the system initiates preemptive heating or cooling to maintain optimal conditions.
The context-aware optimization capabilities of this approach enable thermal strategies to adapt dynamically based on specific driving scenarios. For instance, the system can anticipate increased thermal loads from aggressive driving behaviors or challenging topographical features like steep elevation changes. Beyond simple temperature thresholds, the system incorporates time-based analysis that considers the duration of exposure to suboptimal temperature zones, preventing cumulative thermal damage even when absolute temperatures remain nominally acceptable.
This approach offers multiple advantages over conventional systems. Energy efficiency improves significantly by eliminating unnecessary thermal system activation, directly extending driving range. The personalized control capabilities allow fine-tuning based on individual driver habits, including patterns of infotainment and climate control usage that affect cabin and battery temperatures. Perhaps most importantly, these performance gains come primarily through software intelligence rather than additional hardware components, making the system highly scalable across vehicle platforms.
As connected vehicle technologies continue to advance, these navigation-informed thermal management systems will likely incorporate additional data sources such as vehicle-to-infrastructure communication, real-time weather updates, and crowd-sourced road condition information. This evolution will further enhance predictive accuracy and enable increasingly sophisticated thermal optimization strategies that balance battery preservation, energy efficiency, and performance requirements.
8. Driver Intent and Vehicle State-Based Thermal Management
Electric vehicle battery thermal management has traditionally relied on reactive approaches that activate cooling systems only after temperatures exceed predetermined thresholds. This methodology introduces critical delays in thermal response, particularly during dynamic driving conditions where battery temperatures can rise rapidly. The lag between temperature increase and cooling activation can lead to thermal stress, accelerated degradation, and reduced performance.
Recognizing these limitations, researchers have developed systems that anticipate thermal needs based on driver behavior patterns. One innovative approach uses a fuzzy logic controller to interpret real-time driver inputs—including accelerator and brake pedal movements, vehicle speed, and ambient conditions—to predict imminent thermal loads. The driver intent-based predictive thermal management strategy enables anticipatory cooling through PWM-controlled electronic pumps, reducing thermal lag and maintaining more consistent battery temperatures. This proactive approach is particularly valuable during dynamic driving scenarios involving frequent acceleration or braking, where thermal loads can change rapidly.
Vehicle state and environmental data further enhance thermal control precision. An adaptive thermal management method integrates geographic location, ambient temperature, battery state of charge (SOC), and individual driving habits to select optimal heating or cooling strategies. The system employs threshold mapping tables and data analytics to continuously refine thermal responses based on operating conditions. A dedicated driving habit recognition module personalizes thermal strategies according to individual patterns, improving both energy efficiency and user comfort while adapting to regional climate variations.
For complex scenarios involving multiple competing objectives—such as balancing battery longevity, energy efficiency, and passenger comfort—researchers have developed predictive, optimization-based control systems. One such approach formulates a multi-objective optimization problem that accounts for anticipated driving conditions using a two-stage algorithm to determine optimal power distribution and coolant flow rates. A key innovation in this system is the model linearization through coolant flow update, which transforms the nonlinear thermal system into a computationally manageable form for real-time applications. By integrating predictive data with state estimation techniques, the system achieves enhanced control accuracy while reducing computational demands.
These driver-intent and vehicle-state based approaches represent a significant evolution beyond simple temperature-threshold systems. By anticipating thermal needs rather than merely responding to them, these systems maintain more consistent battery temperatures, reduce thermal stress, and optimize energy usage. As vehicle sensor capabilities and onboard computing power continue to advance, we can expect increasingly sophisticated predictive thermal management systems that further enhance battery performance, longevity, and safety.
9. Machine Learning-Based Optimization of Cooling/Heating Strategies
Traditional thermal management systems for EV batteries typically rely on fixed control algorithms with predetermined temperature thresholds. While functional, these conventional approaches often fail to adapt to the dynamic conditions encountered during real-world driving, resulting in suboptimal energy efficiency and thermal regulation.
Reinforcement learning (RL) has emerged as a powerful alternative to these rigid control strategies. One notable implementation is a reinforcement learning-based thermal management model that uses a Decision Transformer architecture trained on historical driving and thermal data. Unlike conventional systems, this approach learns to predict optimal control actions based on observed battery states and environmental conditions. The model's sophisticated reward function balances temperature regulation against energy consumption from pumps and thermal systems, enabling it to make intelligent trade-offs in real time. By controlling coolant inlet temperature and pump speed simultaneously, the system achieves superior thermal stability while significantly reducing energy usage across diverse operating conditions.
For immersed liquid-cooled battery systems, where thermal sensitivity is particularly high, researchers have developed an AI-powered thermal management system that combines supervised learning for temperature prediction with reinforcement learning for control optimization. This hybrid approach first forecasts future thermal states, then selects the most energy-efficient cooling action based on both current and predicted conditions. The integration of fuzzy logic for control refinement ensures smooth transitions between operating modes, while the modular architecture separates prediction, decision-making, and actuation functions for greater flexibility and scalability. The system's predictive and adaptive control capabilities enable it to anticipate thermal events and apply corrective measures proactively, significantly enhancing battery safety and longevity.
When real-time adaptability to unknown conditions is critical, a Q-learning-based thermal control strategy offers particular advantages. This approach is especially valuable for integrated thermal management across multiple vehicle subsystems, including the battery, motor, and passenger cabin. By continuously learning from real-time data such as vehicle status and power demand, the Q-learning model dynamically adjusts heating power allocation to optimize overall system performance. Its real-time decision-making capabilities ensure effective temperature control even under rapidly changing conditions, contributing to improved energy efficiency and system safety.
A different perspective on machine learning-based thermal optimization focuses on preemptive cooling control. The cooling system using predictive models trains separate models for temperature rise prediction and cooling behavior analysis. By accurately forecasting thermal trajectories, the system determines optimal activation points for cooling systems, avoiding unnecessary operation while maintaining safe temperature limits. This predictive and proactive cooling approach represents a fundamental shift from reactive to anticipatory thermal management, improving both safety margins and operational efficiency.
These machine learning approaches share a common advantage: they can discover complex control strategies that might elude traditional engineering methods, particularly for systems with multiple interacting variables and competing objectives. As battery technologies continue to evolve and fast-charging capabilities advance, these adaptive learning systems will become increasingly valuable for maintaining optimal thermal conditions across diverse operating environments.
10. Charging and Fast-Charging Thermal Management
Fast-charging capabilities have become a key differentiator in the electric vehicle market, but the rapid energy transfer generates substantial heat that must be managed effectively to prevent degradation and safety risks. Traditional thermal management systems, which typically react to temperature thresholds, often struggle to keep pace with the intense thermal dynamics of fast-charging sessions.
To address this challenge, researchers have developed a scene-based thermal management approach that anticipates high-load events such as supercharging. This predictive strategy analyzes vehicle state and environmental conditions to precondition the battery before charging begins, typically by pre-cooling to create thermal headroom. The system employs dual-sided liquid cooling plates supplemented with a cooling fan to ensure uniform temperature distribution across the battery pack. A particularly innovative aspect is its ability to dynamically adjust cooling intensity based on charging phase, reducing or even suspending cooling during the taper (constant voltage) phase when heat generation decreases significantly. This contextual awareness enables more efficient energy usage while maintaining optimal thermal conditions throughout the charging process.
The integration of driver behavior and real-time vehicle feedback represents another significant advancement in charging thermal management. The human-centric predictive thermal control system incorporates driver inputs into the vehicle controller, using simulation models to forecast battery heat generation based on current and anticipated conditions. These predictions undergo continuous correction using real-time battery current data, significantly improving system responsiveness and reliability. The approach orchestrates multiple vehicle subsystems—including engine cooling, motor electronics, air conditioning, and battery cooling—through unified control logic, adjusting electric water pump speeds and fan duty cycles according to predicted thermal loads.
Conventional threshold-based systems often introduce delays in thermal response, as they wait for temperatures to exceed predefined limits before activating cooling mechanisms. The proactive thermal regulation mechanism takes a fundamentally different approach by evaluating real-time parameters—including battery temperature, charge/discharge power, and state of charge—to estimate future thermal trajectories. When the system predicts that continued operation will push the battery outside its optimal temperature range, it initiates thermal management preemptively, reducing thermal stress and improving overall efficiency by avoiding unnecessary heating or cooling cycles.
Resource efficiency represents another critical aspect of charging thermal management. The motor waste heat utilization system enables bi-directional thermal coupling between drive motor and battery circuits, monitoring motor and radiator water temperatures to determine whether motor thermal state can assist in battery temperature regulation. During cold starts, waste heat from the motor warms the battery, while in high-temperature scenarios, the motor circuit can aid in battery cooling. This approach significantly reduces reliance on energy-intensive heating and cooling systems, improving overall efficiency and extending driving range, particularly in cold environments where traditional cooling methods may be less effective.
As fast-charging networks continue to expand and charging rates increase, these advanced thermal management approaches will become increasingly critical for maintaining battery health and safety. The evolution toward predictive, integrated, and resource-efficient thermal control represents a significant step forward in enabling faster charging while preserving battery longevity.
11. Parking and Idle-State Thermal Management
Battery thermal management during parking and idle states presents unique challenges distinct from those encountered during active driving or charging. When a vehicle is parked, particularly after a demanding drive, battery temperatures may remain elevated for extended periods. Conventional systems typically continue cooling until temperatures drop below fixed thresholds, often consuming unnecessary energy and potentially depleting the battery.
To address this inefficiency, researchers have developed a cooling start temperature and time prediction model that leverages historical and real-time vehicle data to build predictive thermal profiles. By analyzing patterns in battery temperature, voltage, and current, the system forecasts temperature trajectories based on route characteristics and ambient conditions. This enables precise determination of when cooling should begin and at what intensity, avoiding premature or prolonged cooling cycles that waste energy. The system incorporates a vehicle-mounted execution module and a data-cleaning pipeline that filters parameters like state-of-charge and mileage to improve modeling accuracy.
A key innovation in this approach is the cloud-vehicle synergy that enables scalable deployment of intelligent thermal control strategies. The cloud component handles complex predictive modeling and parameter optimization, while the vehicle-side system executes the optimized control strategies in real time. This architecture allows for continuous improvement through fleet-wide learning while maintaining responsive local control, ensuring that idle-state cooling remains energy-efficient while keeping the battery within safe operational parameters.
Another significant advancement integrates driver behavior and real-time vehicle conditions into idle-state thermal control logic. Traditional systems often ignore the influence of driver habits and provide limited coordination between thermal subsystems, resulting in suboptimal performance during parking scenarios. The simulation-based prediction and real-time correction mechanism embeds a simulation model within the vehicle controller to predict battery heating based on anticipated conditions. These predictions undergo continuous refinement using real-time sensor feedback, allowing proactive adjustment of cooling actuators such as water pumps and fans.
This integrated approach enhances prediction accuracy while improving actuator responsiveness through dynamic modulation based on heat generation forecasts. The inclusion of driver feedback further personalizes the system's thermal response, ensuring that the battery remains within optimal temperature ranges during idle periods. By coordinating multiple thermal subsystems—including battery cooling, motor electronics, and HVAC—the human-machine interactive thermal management approach provides a comprehensive solution that reduces energy consumption while maintaining thermal stability.
As electric vehicles increasingly incorporate smart connectivity features, these parking and idle-state thermal management systems will likely evolve to incorporate additional contextual information such as weather forecasts, planned departure times from calendar integration, and grid demand signals for intelligent charging coordination. This evolution will further enhance energy efficiency while ensuring that vehicles remain ready for use with batteries at optimal temperatures.
12. Electric-Thermal Coupled Models for Joint SOC and Temperature Estimation
Accurate estimation of battery internal states—particularly state of charge (SOC) and temperature distribution—is fundamental to effective battery management. Traditional approaches often treat these parameters separately, despite their significant interdependence. SOC estimation methods like open-circuit voltage mapping and equivalent circuit models typically require long relaxation times and show parameter sensitivity under dynamic conditions. Similarly, conventional temperature estimation techniques based on impedance spectroscopy or complex thermal models frequently struggle with real-time implementation and require extensive sensor arrays.
To overcome these limitations, researchers have developed an electric-thermal-neural network (ETNN) coupling model that integrates electrical and thermal networks with data-driven neural networks. This unified approach enables joint estimation of SOC and state of temperature (SOT) across diverse operating conditions. By modeling the nonlinear interactions between electrical and thermal behaviors, the system achieves enhanced estimation accuracy, particularly under extreme conditions such as high-current operations or temperature extremes. The reduced reliance on physical sensors lowers system complexity and cost while maintaining or even improving estimation performance.
Thermal safety during high-rate discharges presents particular challenges, as surface temperatures can increase rapidly and potentially trigger thermal runaway. Conventional neural networks often exhibit limitations in this context, including slow convergence and poor generalization to new conditions. A wavelet neural network (WNN) optimized via simulated annealing (SA) addresses these challenges by leveraging the time-frequency localization properties of wavelets to capture transient thermal behaviors. The SA optimization enhances convergence and helps avoid local minima during training, resulting in more accurate forecasting of hot spot temperatures based on discharge current and ambient conditions.
The distributed nature of modern vehicle architectures creates opportunities for more sophisticated thermal control approaches. A distributed AI-powered cooling control system deploys server-based predictive models across vehicle fleets, with individual vehicles acting as client units that collect operational and temperature data in real time. These client units use server-supplied models to forecast component temperatures and activate cooling mechanisms proactively when critical thresholds are predicted. This architecture improves prediction accuracy through continuous learning while reducing dependence on external data sources, ensuring reliable thermal management across diverse driving scenarios.
These electric-thermal coupled models represent a significant advancement beyond traditional approaches that treat electrical and thermal behaviors as separate domains. By recognizing and modeling the complex interactions between these aspects, these systems achieve more accurate state estimation, better thermal prediction, and more effective control strategies. As battery technologies continue to evolve toward higher energy densities and faster charging capabilities, these integrated approaches will become increasingly valuable for maintaining safety and performance across diverse operating conditions.
13. Classical Machine Learning Models for Safety and Thermal Risk Assessment
While deep learning approaches have gained significant attention in battery thermal management, classical machine learning techniques continue to offer valuable solutions, particularly for safety-critical applications where interpretability and computational efficiency are paramount.
Uneven thermal distribution in battery packs represents a persistent challenge that can lead to accelerated degradation and safety risks. The electrochemical-thermal coupling (ECT) model addresses this by integrating electrochemical behavior with thermal modeling to predict and mitigate issues such as lithium plating and thermal non-uniformities. Traditional cooling systems often inadequately address temperature gradients within battery packs, resulting in hotspots and uneven current distribution. The ECT model generates detailed thermal maps reflecting real-time usage patterns and environmental conditions, enabling region-specific thermal control through localized cooling or heating. This approach not only extends battery life by minimizing parasitic reactions like solid-electrolyte interphase (SEI) growth but also enhances safety by preventing dendritic growth and internal shorts during high-rate charging.
Cold-weather operation presents unique challenges for EV batteries, including increased impedance and reduced energy density. The integration of intelligent networked vehicle data with model predictive control (MPC) offers significant advantages over conventional heating methods such as air or liquid convection. This system leverages real-time and predicted vehicle speed data to optimize heating strategies, modeling battery load current as a function of traction power to adjust compressor and pump speeds accordingly. The MPC framework dynamically tailors thermal inputs to driving conditions, reducing energy consumption while maintaining optimal temperature ranges. This approach is particularly valuable for intelligent networked vehicles, where additional data sources can further enhance predictive accuracy.
Energy efficiency remains a critical concern in battery thermal management, with traditional PID or rule-based systems often employing conservative control margins that waste energy. The Advanced Model Predictive Control (AMPC) framework addresses this through a software-driven solution that optimizes temperature control without requiring additional hardware. The system uses a multi-model architecture combining quasi-static gray-box modeling, lumped parameter methods, and heat generation models to predict battery and coolant temperatures. An optimization algorithm continuously evaluates parameter sets for temperature accuracy and energy consumption, updating them based on real-time feedback. The implementation of Linear Quadratic Programming (LQP) ensures low computational overhead, enabling real-time responsiveness even with limited computing resources.
These classical machine learning approaches demonstrate that sophisticated thermal management doesn't necessarily require deep neural networks or extensive computational resources. By combining physical understanding with targeted machine learning techniques, these systems achieve robust performance while maintaining interpretability and computational efficiency—qualities particularly valuable in safety-critical applications where understanding model behavior is essential for validation and certification.
14. Cold-Weather and Preconditioning Strategies
Cold-weather operation presents some of the most significant challenges for electric vehicle batteries. Low temperatures increase internal resistance, reduce power output, and elevate safety risks such as lithium plating during charging. Traditional heating methods like positive temperature coefficient (PTC) heaters consume substantial energy, significantly impacting vehicle range. These challenges have driven the development of innovative approaches to cold-weather battery management.
One significant advancement integrates intelligent networked information with predictive control frameworks. The integration of intelligent networked information introduces a model predictive control (MPC) system that leverages predicted vehicle speed data to optimize battery heating in cold environments. By dynamically adjusting compressor and pump speeds based on anticipated driving conditions, the system improves heat transfer efficiency and enables faster, more uniform battery warming. The incorporation of a load current model tied to vehicle power demand ensures that thermal control responds appropriately to both current and forecasted operational states, enhancing safety while minimizing energy consumption.
Balancing passenger comfort with battery thermal needs presents another challenge in cold weather. The cooperative heating control method employs distributed model predictive control (DMPC) to simultaneously optimize thermal conditions for both the passenger cabin and the battery. This approach decomposes the vehicle's thermal system into two subsystems—cabin and battery—each managed by an independent MPC controller. The controllers communicate using a cooperative strategy, allowing real-time adjustments based on sensor feedback, environmental conditions, and user preferences. The integration of a heat pump with auxiliary PTC heating reduces overall energy consumption while maintaining both thermal comfort and battery performance.
Geographic location significantly influences optimal thermal management strategies. The intelligent thermal management system introduces a context-sensitive approach that adjusts heating strategies based on geographic latitude, local ambient temperature, battery temperature, and state of charge. Using threshold mapping tables customized for different environmental conditions and driving habits, the system selects the most appropriate heating or cooling mode for each situation. This location-aware approach is particularly valuable in regions with harsh winters, where optimized thermal strategies can significantly improve range and performance.
Battery aging introduces additional complexity to cold-weather thermal management. The adaptive thermal management system addresses this by adjusting temperature thresholds based on the battery's state of health (SOH). Unlike conventional systems that apply static thermal limits regardless of battery age, this approach introduces a multi-level regulation mechanism that dynamically modifies thermal thresholds to prioritize either efficiency or battery preservation depending on the current SOH. In cold-weather conditions, where thermal stress is already elevated, this adaptive strategy ensures that aging batteries receive appropriate thermal protection without unnecessarily compromising energy efficiency.
These advanced cold-weather strategies represent significant progress beyond traditional heating approaches, offering more energy-efficient, context-aware, and adaptive solutions for maintaining battery performance and longevity in challenging thermal environments. As electric vehicles continue to penetrate markets with extreme climates, these sophisticated preconditioning and thermal management approaches will become increasingly essential for ensuring consistent performance and user satisfaction across all operating conditions.
Get Full Report
Access our comprehensive collection of 266 documents related to this technology
Identify Key Areas of Innovation in 2025
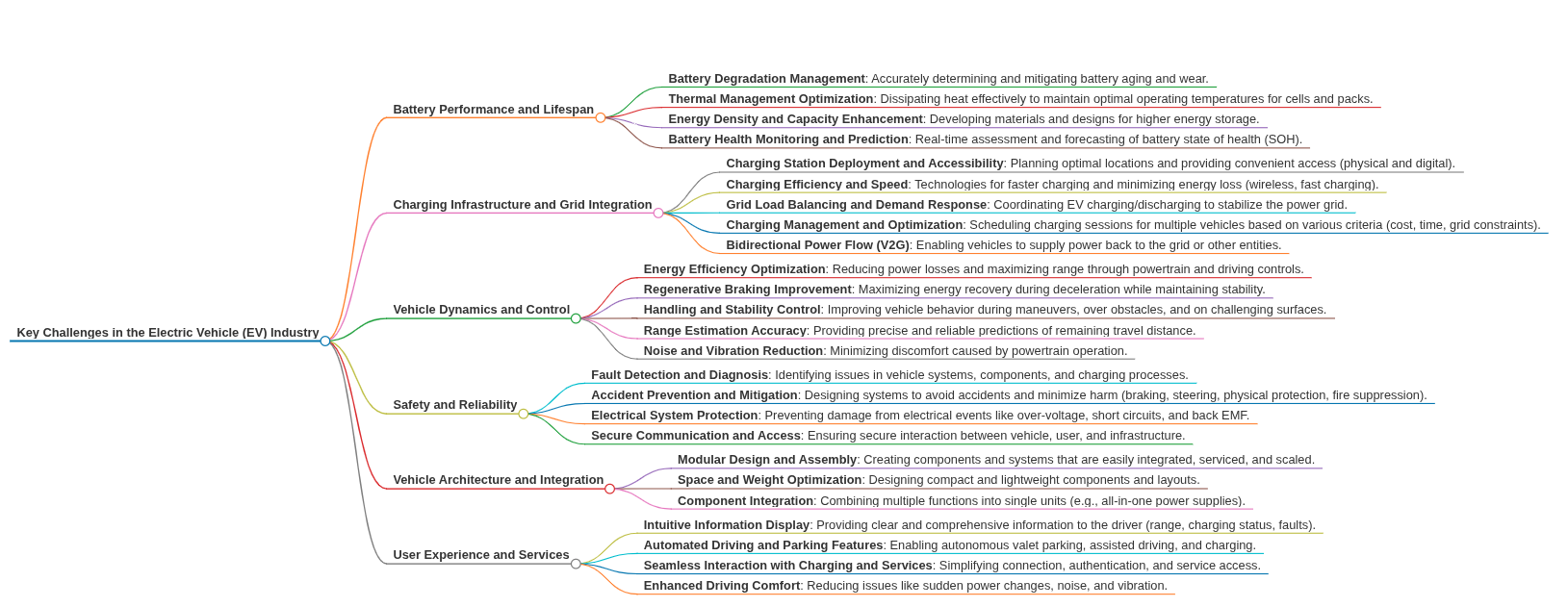