Deviation Control in EV Battery Production
Manufacturing variability in EV battery production manifests across multiple scales - from microscopic electrode coating inconsistencies to millimeter-scale welding defects. Current production data shows that even minor deviations in cell assembly can lead to capacity variations of 2-5% between otherwise identical cells, while welding imperfections account for up to 15% of cell connection failures in battery modules.
The fundamental challenge lies in maintaining precise control over hundreds of process parameters while scaling production to meet growing demand for electric vehicles.
This page brings together solutions from recent research—including adaptive laser welding systems that compensate for surface variations, simplified busbar designs that reduce assembly complexity, standardized cell architectures that enable scalable manufacturing, and improved sealing techniques that enhance reliability. These and other approaches focus on reducing variability while maintaining high throughput in mass production environments.
1. Jig Assembly with Tilting Mechanism for Height Compensation in Laser Welding Battery Cell Leads
LG Chem, Ltd., 2021
Jig assembly for laser welding battery cell leads that compensates for height deviations and improves welding quality. The jig has a tilting mechanism with a hinge and spring that allows the welding contact member to tilt and maintain contact with the cell lead surface even if it is not flat or uniform. This compensates for height deviations and ensures continuous contact between the cell lead and laser for consistent welding quality.
2. Machine Learning-Driven Monitoring and Adjustment System for Battery Manufacturing Parameters
HONEYWELL INTERNATIONAL INC., 2024
Autonomous optimization of battery manufacturing processes using machine learning. The method involves monitoring battery manufacturing parameters and generating deviation events when thresholds are exceeded. These deviation events are compared to historical events using similarity analysis. If similar, the historical adjustments are used for the new event. If dissimilar, machine learning models predict outcomes for adjustment candidates. Thresholds on confidence and risk determine autonomous vs supervised adjustment.
3. Process Control System with Predictive Adjustment for Non-Aqueous Electrolyte Secondary Battery Manufacturing
PRIMEARTH EV ENERGY CO LTD, 2024
Process control system for manufacturing non-aqueous electrolyte secondary batteries that prevents quality degradation without waiting for completed battery evaluation. The system calculates predicted cell characteristics based on material properties and manufacturing conditions. It then adjusts process targets at each step to bring the predicted characteristics closer to ideal values. This proactive correction aims to prevent quality issues during the battery manufacturing process itself, rather than waiting for finished battery evaluation.
4. Battery Cell Sorting Method and Device Utilizing Historical Data-Based Model for Quality Assessment
ELIIY POWER CO LTD, 2023
Battery cell sorting method and device that accurately determine good or defective cells at each step of production, monitoring, and handling to improve yield, reduce waste, and optimize cell reuse. The method involves creating a model to distinguish good from bad cells based on extracted regularities from historical cell data at each step. This model is used to determine cell quality at each step. For example, after initial testing, a model may identify singular points between good and bad cells based on charge/discharge characteristics. This model is then used to determine if a cell is good or bad after charging/discharging. This allows more efficient sorting compared to full tests at each step. The method also involves selectively aging cells that are initially classified as good but have high self-discharge rates.
5. Battery Production Prediction System Utilizing Historical Monitoring Data for Quality Assessment and Proactive Adjustment
WUXI LEAD INTELLIGENT EQUIPMENT CO LTD, 2023
Predicting and planning battery production to improve yield and stability. It involves using monitoring data from previous batteries to forecast the production quality of the next battery. The forecast is compared against threshold values to determine if the next battery will be normal, abnormal, or require correction. This allows proactive adjustments to avoid issues and improve yield. If abnormal, it also generates early warning to address issues.
6. Battery Production System with Real-Time Adaptive Process Parameter Adjustment Based on Sensor and Quality Metric Correlation
SIEMENS AG, SIEMENS AKTIENGESELLSCHAFT, 2023
Optimizing battery production to reduce defect rates by dynamically adjusting process parameters based on real-time measurements and cell quality. Sensors monitor production parameters like temperature, humidity, and voltage. Cell quality metrics like self-discharge rate, capacity, and internal resistance are measured. By correlating these measurements, controllable process parameters are identified that impact cell quality. The production process is then adjusted in real-time to optimize cell quality. This adaptive process control reduces defect rates compared to fixed parameter settings.
7. Method for Analyzing Lithium-Ion Battery Performance Variability Using Statistical Models and Visualization Techniques
Guolian Automotive Power Battery Research Institute Co., Ltd., CHINA AUTOMOTIVE BATTERY RESEARCH INSTITUTE CO LTD, 2023
Predicting lithium-ion battery performance inconsistency caused by manufacturing process variations to enable better control of battery design and consistency. The method involves analyzing the relationship between battery performance and process parameters using statistical models. By collecting design parameters and process deviations, consistency is analyzed using contour maps and probability cloud images. This provides intuitive visualization of how process variations affect performance and allows calculation of the probability distribution of performance consistency. It enables predicting battery performance inconsistency levels based on process variability and guiding measures to reduce deviations.
8. Statistical Model for Predicting Inconsistency in Lithium-Ion Battery Performance from Manufacturing Variations
Guolian Automotive Power Battery Research Institute Co., Ltd., CHINA AUTOMOTIVE BATTERY RESEARCH INSTITUTE CO LTD, 2023
Predicting the inconsistency of lithium-ion battery performance due to manufacturing variations using a statistical model. The method involves breaking down battery performance parameters into local parameters at multiple levels. It then calculates the inconsistency distribution of each local parameter using statistical models based on the manufacturing process variables. By combining these local inconsistencies, it predicts the overall inconsistency of battery performance parameters. The models consider independence, matching, and correlation between process variables to accurately represent inconsistency propagation.
9. Battery Manufacturing Process with Machine Learning-Driven Predictive Modeling and Parameter Optimization
PRIMEARTH EV ENERGY CO LTD, 2023
Optimizing battery manufacturing processes using machine learning and optimization techniques to improve battery performance and yield. The method involves generating predictive models of control index values based on material and manufacturing conditions using machine learning. It then uses optimization to determine the optimal values for the controllable parameters that minimize variations in the control index values. This allows automated optimization of critical parameters during battery production without needing precise control of non-controllable conditions.
10. Manufacturing System for Battery Cells with Automated Real-Time Parameter Adjustment
NINGBO VEKEN BATTERY CO LTD, 2021
Intelligent manufacturing method and system for battery cells that enables automated adjustment of real-time process parameters during battery production to improve yield and quality. The method involves setting and saving process parameters based on cell model, then intelligently correcting real-time equipment parameters that drift outside the set range. This prevents manual parameter resetting when swapping cells and allows real-time parameter adjustment during production. The method uses a manufacturing execution system to monitor equipment parameters, determine if they're within limits, calculate correction values, and feed back adjustments to the equipment.
11. Battery Module with Zigzag Electrode Tabs for Enhanced Weld Joint Stability
SK Innovation Co., Ltd., 2018
Battery modules for electric vehicles and grid storage that are easier to assemble and have improved weld joint reliability. The module uses a zigzag bending pattern for the electrode tabs that connect the battery cells. This zigzag shape absorbs vibrations during ultrasonic welding, preventing damage to the cells.
12. Battery Cell Terminal with Thinner Cross-Section for Stress-Reduced Laser Welding of Current Collector Foils
TOYOTA JIDOSHA KABUSHIKI KAISHA, 2017
Reducing damage to battery cell current collectors during welding to improve overall cell performance and reliability. The method involves designing the battery cell terminal to have an extended portion with a thinner cross-section. When the current collector foils are laser welded to the terminal, the thinner section and protruding foils are fused. This reduces the volume of molten metal that solidifies and shrinks during cooling, avoiding stresses that can tear the foils.
13. Predictive Control System with Adaptive Mathematical Models for Manufacturing Process Variation Management
OMRON CORP, OMRON TATEISI ELECTRONICS CO, 2017
Predictive control system for optimizing manufacturing processes and product quality by avoiding accumulation of variations. The system predicts product characteristic values during manufacturing using mathematical models based on past data and current process values. If the predictions deviate from targets, it calculates optimum process conditions to correct the deviation. This allows active control to reduce product variation by compensating for accumulated errors. The models are periodically updated based on measured data to adapt to process changes.
Get Full Report
Access our comprehensive collection of 13 documents related to this technology
Identify Key Areas of Innovation in 2025
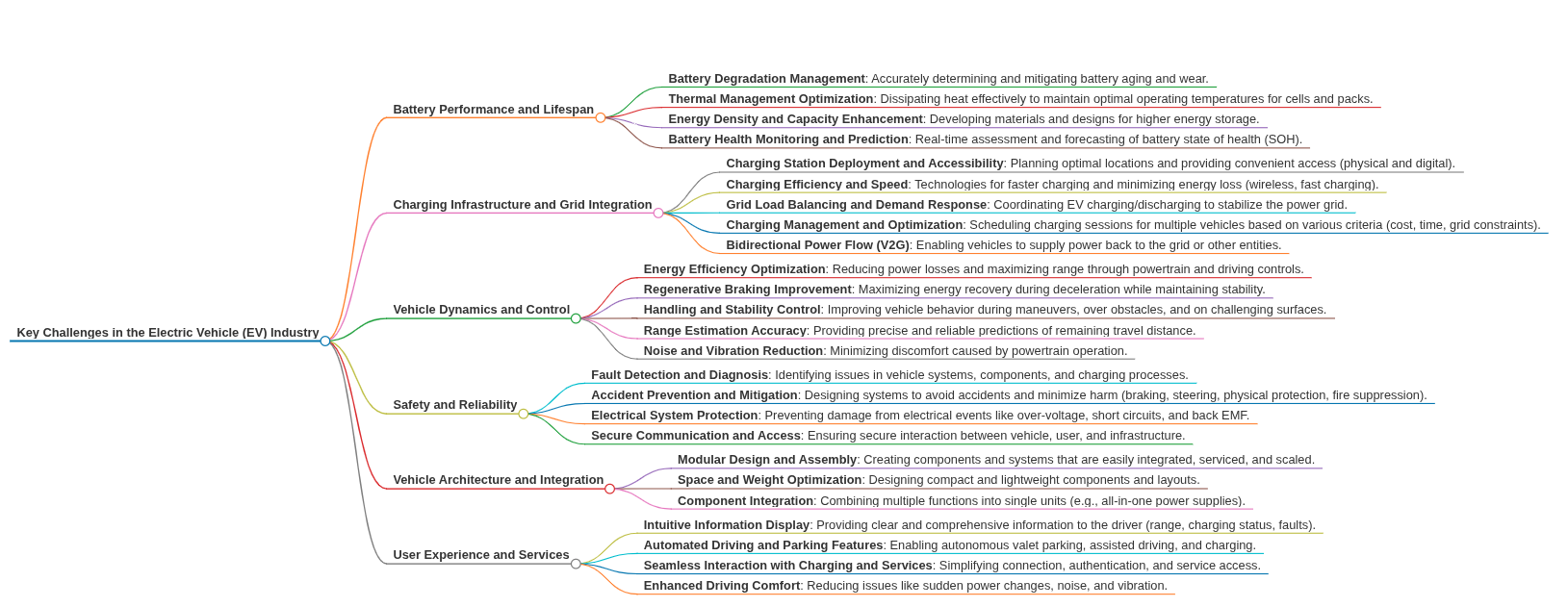